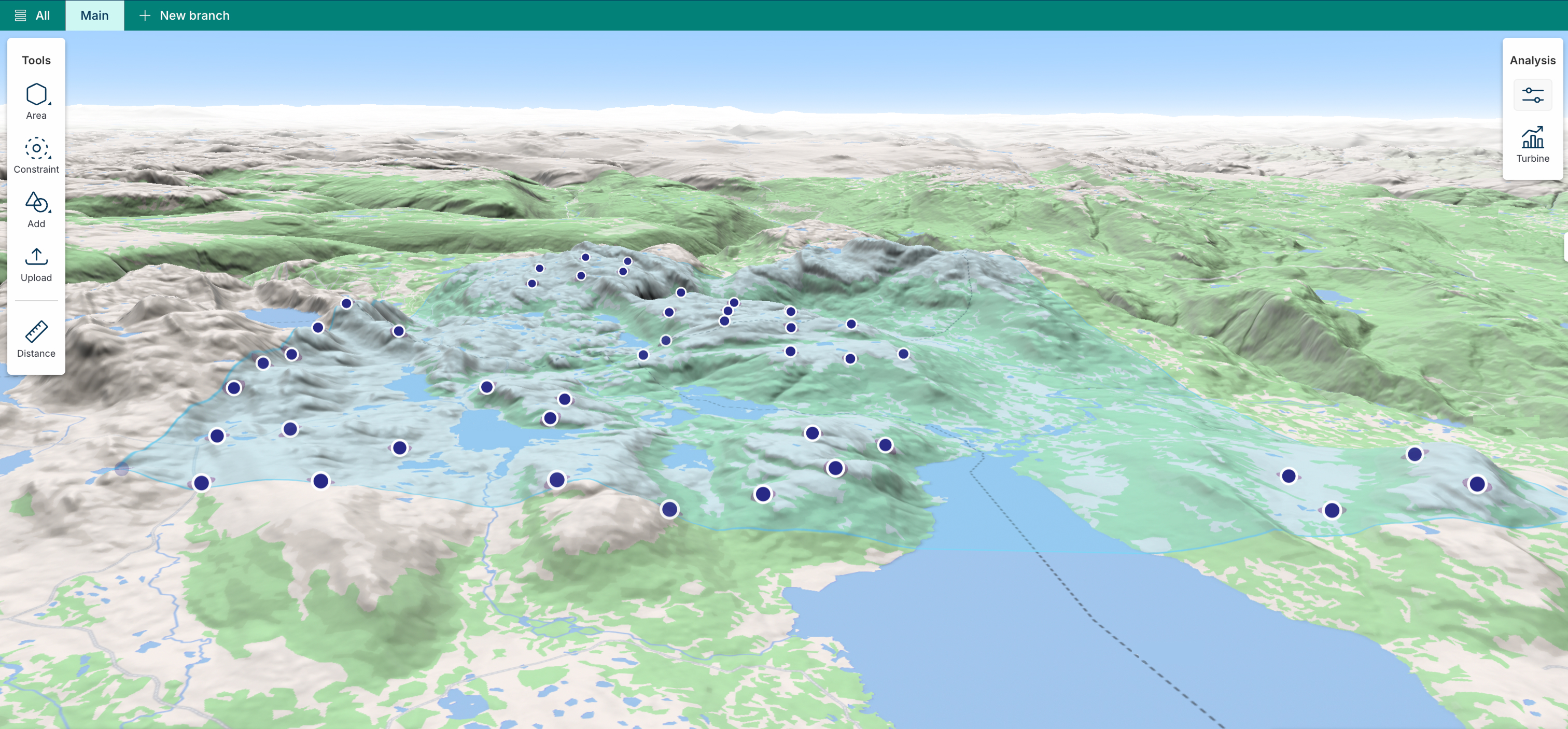
Wind farms being developed today are bigger, more constrained, and increasingly complex.
In a fast-changing environment, finding the optimal turbine layout requires rapid design iterations, the ability to explore a wide range of “what if” scenarios with high accuracy, and responding quickly when assumptions change.
Traditionally, the wind industry relies heavily on gradient-free optimisation methods. The benefit of these methods is that they can handle non-differentiable functions and integrate more easily with existing wake models. But these methods don’t scale. For modern wind farms, with thousands of design variables, they quickly become computationally infeasible. This slows down design iterations and limits how thoroughly assumptions can be tested, increasing the risk of settling for a suboptimal layout.
We knew that it was possible to create a new type of optimisation engine. Where even for large wind farms, project teams could model the site as it truly is, accounting for real-world wind variations, complex site constraints, and thousands of design variables, without cutting any corners to make the computation work. Optimisation that required no compromise between accuracy and feasibility, so that project teams could ground their decisions in accurate models and up-to-date information.
“Our goal was to create an optimisation engine that optimises wind farm layouts in minutes,” explains Senior engineer and PhD John Marius Hegseth, the mind behind the Vind AI optimisation engine.
This is exactly what we have done with the Vind AI optimisation engine, by using a combination of state-of-the-art optimisation techniques and innovative analysis methods.”
“Our goal was to create an optimisation engine that optimises wind farm layouts in minutes.”
John Marius Hegseth, Senior Algorithm Engineer, Vind AI
Our optimisation engine uses gradient-based optimisation with analytic gradients.
While this approach requires differentiable functions and additional implementation effort, it unlocks higher accuracy and drastically improved computational efficiency.
Our Vind AI optimisation engine is built on extremely fast, fully differentiable models for the annual energy production, constraints, and even LCoE calculations, combined with their analytic derivatives. The engine’s speed and accuracy is made possible by incorporating several novel analysis methods (and developing some of them further ourselves in the process!). Using a novel model for the yield assessment is a particularly important feature of our optimisation engine, since this is what typically becomes a bottleneck when optimising large wind farm layouts.
This enables efficient layout optimisation, even for large wind farms with complex constraints and local wind variations.
“For large-scale wind farm design, onshore and offshore, this is the only method that truly scales,” explains John Marius.
“For large-scale wind farm design, onshore and offshore, this is the only method that truly scales.”
John Marius Hegseth, Senior Algorithm Engineer, Vind AI
johnmarius@vind.ai